Developed to date models predicting the spread of epidemic diseases, and based on them decision support systems using dynamic compartment of models of populations with global mixing, which does not allow to simulate the spatial distribution of diseases. It is also known to develop using graphs human settlements and traffic flows, but they are not adequately describe the processes of the spread of diseases transmitted through the environment and through the migration of carriers of infection [8, 9].
There are a number of mathematical models of parallel and distributed computing to solve problems on the spatial distribution of epidemics. One of them is cellular automata. CA are discrete dynamical systems whose behavior can be completely described in terms of local dependency, they can be used to describe the processes of continuous dynamic systems, such as population with flowing epidemic disease [10-13].
The epidemiological situation, in accordance with the data, corresponds to the classical SIR model. As the first step in the modeling process, we identify the independent and dependent variables. The independent variable is time t, measured in days. In one of the first epidemic models that describe three population groups: the number of susceptible individuals (S), the number of infected individuals (I), and recovered with long-term immunity (R) [14].
The proposed method of spatial spread of influenza modeling in spatially distributed populations is to use the combined simulation model based on stochastic models with the assumption of a global mixing of individuals, and two-dimensional cellular automata. In the simulation of migration flows and mixing population, based on cellular automata between elementary populations, migration happens. The selection of individuals for migration may take place as follows: Migration intensity is constant for the entire population; migration occurs uniformly from all groups [15, 16].
Figure 1 shows the SIR model of an epidemic disease based on a stochastic compartment of model using cellular automaton [17, 18].
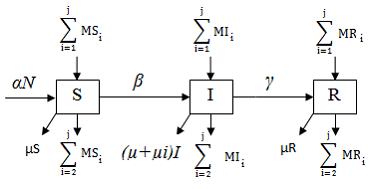
Fig. 1. Proposed SIR model.
When modeling migration flows and population mixing based on a cellular automaton, the SIR model is defined by the system. Differential equations of the flu distribution model (at Δt = 1):
(1)
St - is the number of susceptible individuals in the population at time t, It - is the number of infected individuals in the population at time t, Rt - is the number of recovered individuals in the population at time t. rb – is the operator of setting a random variable in accordance with the binomial distribution law. If rb = Randbinom (p; n), then p is the number of intensity, probability of success, n is the number of tests [19].
When modeling the SIR model using deterministic and stochastic models for the comparative analysis, shows that in the modeling deterministic processes there the absence of any random effects, and which get one result inevitable. While, in the modeling stochastic process (random) can lead to get different results with a certain prospect. This suggests that the use of stochastic models can get the most accurate and relevant results for a real spread of the epidemic [20-22].
Description parameters of the SIR-model are shown in table 1.
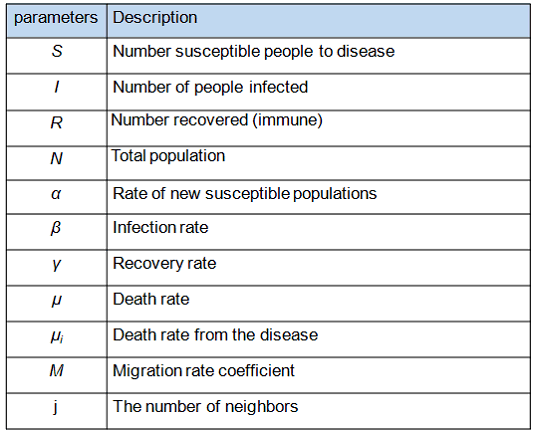
At realization the model using computer graphics simulation Any Logic program [23, 24] transition graph simulation model of influenza as shown in figure 2.
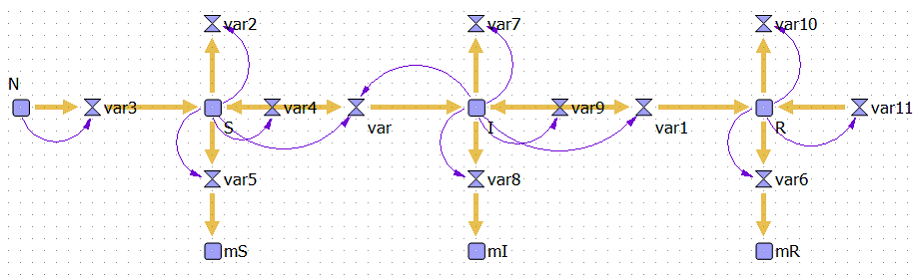
Fig. 2 Simulation model using package AnyLogic.
AnyLogic supports the development and modeling of feedback systems (flow and accumulator diagrams, decision rules, including arrays of variables). The model developed in the Any Logic environment is designed to study the characteristics of an epidemic and the system recovery process. The environment allows you to change the values of the model parameters directly during its operation, which in real life is similar to human intervention in various processes [25-29].
To obtain the results when modeling the SIR model using cellular automaton for the predictive assessment of the spatial spread of epidemic diseases, the following values of the system parameters β = 0.002, γ = 0.5, M= 0.001. The solutions found in the time interval [0-15] day.
Figures 3, 4 shows the sensitivity of the comparative study for susceptible people to disease (S) and number of people infected (I) between compartment simulation model and the proposed model using cellular automaton of the spread of influenza to a change in the migration rate coefficient (M) in the time interval [0-15] days.
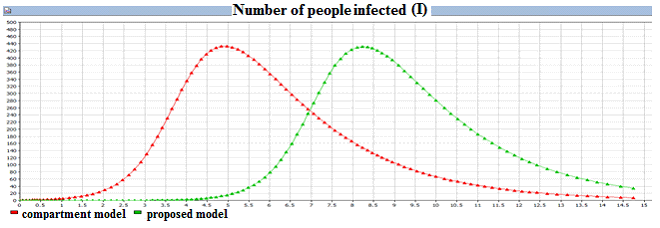
Fig. 4 comparative study for number of people infected.
In figures 3, 4 there is a change in the process of spread of influenza in the population depending on the migration rate coefficient (M). As follows from the graphs, the value of this parameter affects the nature of the development of the simulated epidemic process [30].
When modeling migration flows and mixing populations based on cellular automata between elementary populations that correspond to cells, migration occurs. The selection of individuals for migration can occur as follows: the intensity of migration is constant for the entire population; migration occurs from all groups evenly [31- 32].
The results of the comparative analysis of the model indicate that the decrease in the intensity of migration flows (M) between the compartments leads to delay the spread of epidemic (the number of infections) compared with models with global mixing and allows you to quickly (operatively) control and analyze the patterns of spread of disease, thus, increase the quality of management of anti-epidemic work.
References
- Башабшех, М. М., Масленников, Б. И., & Скворцов, А. В. (2013). Комбинированная имитационная модель пространственного распространения эпидемических заболеваний по холере на основе вероятностного клеточного автомата. Интернет-журнал Науковедение, (3), 47-47.
- Башабшех, М.М., Масленников, Б.И., Скворцов, А.В. Комбинированная имитационная модель пространственного распространения эпидемических заболеваний по холере на основе вероятностного клеточного автомата//Интернет-журнал «Науковедение». 2013 №3 (16) [Электронный ресурс].-М. 2013. – Режим доступа: http://naukovedenie.ru/PDF/42tvn313.pdf.
- Bashabsheh, M. M., Maslennikov, B. I., & Skvorcov,A. V. (2013). Kombinirovannaja imitacionnaja model’prostranstvennogo rasprostranenija jepidemicheskih zabolevanij po holere na osnove verojatnostnogo kletochnogo avtomata. Internet-zhurnal «Naukovedenie, (3), 16.
- Башабшех, М. М., & Масленников, Б. И. (2013). Имитационное моделирование пространственного распространения эпидемий (на примере холеры) с применением метода клеточных автоматов с помощью программы AnyLogic. Интернет-журнал Науковедение, (6), 127-127.
- Башабшех, М.М., Масленников, Б.И. Имитационное моделирование пространственного распространения эпидемий (на примере холеры) с применением метода клеточных автоматов с помощью программы AnyLogic//Интернет-журнал «Науковедение». 2013 №6 (19) [Электронный ресурс]. -М. 2013. – Режим доступа: http://naukovedenie.ru/PDF/135TVN613.pdf.
- Bashabshekh, M. M., & Maslennikov, B. I. (2013). Simulation modeling of the spatial spread of epidemics (cholera for example) using the method of cellular automatausing the Anylogic. Naukovedenie, (6).
- Башабшех, М. (2014). Комбинированная имитационная модель пространственного распространения эпидемий на основе стохастической компартментной модели и вероятностного клеточного автомата (Doctoral dissertation, Юго-Зап. гос. ун-т).
- Башабшех, М. М., & Масленников, Б. И. (2012). Компартментные модели распространения заболеваний (эпидемии). In Система гарантий качества образования: Разработка и внедрение: материалы научнопрактической конференции.–Тверь: Купол (pp. 23-27).
- Башабшех, М. М., Скворцов, А. В., & Масленников, Б. И. (2013). Исследование и прогнозирование эпидемиологических заболеваний на основе компартментальных моделей. Сборник научных трудов магистрантов и аспирантов. Раздел «Информационные технологии в науке и образовании». Тверь: ТвГТУ, (3), 6.
- Башабшех, М. М., Скворцов, А. В., & Масленников, Б. И. (2013). Имитационное моделирование пространственного распространительных динамических систем с использованием вероятностных клеточных автоматов на основе регулярных гексагональных решёток. In Образование и наука: современное состояние и перспективы развития (pp. 25-26).
- Башабшех М.М., Скворцов А.В., Масленников Б.И. Совмещение вероятностных клеточных автоматов и компартментных моделей для прогнозной оценки пространственного распространения эпидемиологических заболеваний. // Сб. Трудов НТК. Конференции: «Интеграция науки и образования-производству, экономике» , 12 декабря 2012. Tом 2. С10. Тверь.
- Башабшех, М. М., Скворцов, А. В., & Масленников, Б. И. (2012, December). Совмещение вероятностных клеточных автоматов и компартментных моделей для прогнозной оценки пространственного распространения эпидемиологических заболеваний. In Сб. Трудов НТК. Конференции:«Интеграция науки и образования-производству, экономике (Vol. 12).
- Bashabsheh, M. M., Skvorcov, A. V., & Maslennikov, B. I. Sovmeshhenie verojatnostnyh kletochnyh avtomatov i kompartmentnyh modelej dlja prognoznoj ocenki prostranstvennogo rasprostranenija jepidemiologicheskih zabolevanij. Sb. Trudov NTK. Konferencii:«Integracija nauki i obrazovanija-proizvodstvu, jekonomike, 12.
- Kermack W.O. A Contribution to the Mathematical Theory of Epidemics. / A.G. McKendrick, W.O. Kermack //Proc. Roy. Soc. Lond. A 115. 1927. C.700-721.
- Башабшех М.М., Скворцов А.В., Масленников Б.И. Применение клеточных автоматов для моделирования пространственного распространения эпидемиологических заболеваний. // Вестник тверского государственного технического университета: Научный журнал.Тверь:ТвГТУ. №1, 2013. Вып.23. 9 с.
- Башабшех, М. М., Скворцов, А. В., & Масленников, Б. И. (2013). Применение клеточных автоматов для моделирования пространственного распространения эпидемиологических заболеваний. Вестник тверского государственного технического университета: Научный журнал. Тверь: ТвГТУ, (1).
- Башабшех, М. М., Скворцов, А. В., & Масленников, Б. И. (2013). Применение клеточных автоматов для моделирования пространственного распространения эпидемиологических заболеваний. Вестник Тверского государственного технического университета, (1), 9-13.
- Башабшех, М. М., Скворцов, А. В., & Масленников, Б. И. ПРИМЕНЕНИЕ КЛЕТОЧНЫХ АВТОМАТОВ ДЛЯ МОДЕЛИРОВАНИЯ ПРОСТРАНСТВЕННОГО РАСПРОСТРАНЕНИЯ ЭПИДЕМИОЛОГИЧЕСКИХ ЗАБОЛЕВАНИЙ.
- Башабшех, М. (2014). Комбинированная имитационная модель пространственного распространения эпидемий на основе стохастической компартментной модели и вероятностного клеточного автомата: специальность 05.13. 01″ Системный анализ, управление и обработка информации (по отраслям) (Doctoral dissertation, автореферат диссертации на соискание ученой степени кандидата технических наук/Башабшех Мурад).
- Башабшех, М. М., Скворцов, А. В., & Масленников, Б. И. (2013). Исследование пространственно распределенных динамических систем при моделировании распространения эпидемических заболеваний методами вероятностного клеточного автомата. Перспективы науки, (5), 60-63.
- Башабшех, М. М. (2014). Математическое моделирование распространения эпидемий (на примере холеры) с использованием детерминированной и стохастической компартментных моделей. In Перспективы развития науки и образования (pp. 15-16).
- Башабшех, М. М., Скворцов, А. В., & Масленников, Б. И. (2013). Моделирование пространственного распространения эпидемии с использованием регулярных гексагональных решёток на основе вероятностных клеточных автоматов. Вестник ТвГТУ, 84(23,№ 1), 28-32.
- Karpov Ju. G. Imitacionnoe modelirovanie sistem. Vvedenie v modelirovanie s AnyLogic 5. — SPb: BHV-Peterburg, 2006. — 400 s. — ISBN 5-94157-148-8.
- Using environment Any Logic for modeling distribution of epidemic // Modern scientific researches and innovations. 2013. № 4 [Electronic journal]. URL: http://web.snauka.ru/en/issues/2013/04/23264
- Башабшех, М. М. (2013). Использование среды Anylogic при моделировании распространения эпидемии. Современные научные исследования и инновации, (4), 2-2.
- Башабшех, М. М., Масленников, Б. И., & Скворцов, А. В. (2015). Программа для прогнозирования пространственно-временного распространения эпидемий с использованием метода клеточного автомата.
- Башабшех, М. М. (2015). Разработка имитационной модели распространения эпидемий на основе вероятностного клеточного автомата. Вестник компьютерных и информационных технологий, (1), 6-9.
- Махмуд, Б. М. (2014). Повышение качества и точности противоэпидемической ситуации с применением комбинированной имитационной модели на основе стохастической компартментной модели и клеточного автомата. Инженерный вестник Дона, 28(1), 58.
- Башабшех, М. М. (2013). Повышение качества и точности противоэпидемической ситуации с применением комбинированной имитационной модели на основе стохастической компартментной модели и клеточного автомата.
- Скворцов, А. В., & Башабшех, М. М. (2013). ПРИМЕНЕНИЕ ДИНАМИЧЕСКИХ СИСТЕМ, ИСПОЛЬЗУЮЩИХ МЕТОД ВЕРОЯТНОСТНОГО КЛЕТОЧНОГО АВТОМАТА ПРИ ИМИТАЦИОННОМ МОДЕЛИРОВАНИИ ПРОЦЕССА РАСПРОСТРАНЕНИЯ ЭПИДЕМИИ ХОЛЕРЫ. Журнал научных публикаций аспирантов и докторантов, (4), 226-228.
- Махмуд, Б. М., Масленников, Б. И., & Скворцов, А. В. (2013). Комбинированная имитационная модель пространственного распространения эпидемических заболеваний по холере на основе вероятностного клеточного автомата. Вестник евразийской науки, (3 (16)), 47.
- БАШАБШЕХ, М.,СКВОРЦОВ,А.,&МАСЛЕННИКОВ,Б. ВЕРОЯТНОСТНОГО КЛЕТОЧНОГО АВТОМАТА. ПЕРСПЕКТИВЫ НАУКИ, 60.